Description
Big Data in Astronomy
Scientific Data Processing for Advanced Radio Telescopes
Coordinators: Kong Linghe, Huang Tian, Zhu Yongxin, Yu Shenghua
Language: English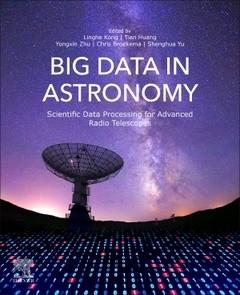
Subjects for Big Data in Astronomy:
Keywords
21cm line; Algorithm reference library; Application design; Architecture; ARL image library; Artificial intelligence; ASIC; Astronomical big data; Astronomical data analysis; Astronomy; BaSC; Big data; Calibration; Channelization; Computation topology model; Correlation; Dark age; Dask; Data driven; Data pipelines; Data redistribution; Data reduction; Data transmission model of processors; Deconvolution; Digital signal processing; Digitization; Dirty map; Distributed computing; Execution frame; Execution framework; Five-hundred-meter aperture spherical radio telescope; Flux; Foreground; FPGA; Gain; GPU; Gridding; Heterogeneous; High-performance computing; Image recognition; Image-plane analysis; Interferometry; Large-scale structure; Least-misfit gridding function; Manycore; Mid-frequency aperture arrays; Morphological classification of galaxies; Neutral hydrogen; Packet capture; Packeting; Performance optimization; Periodicity analysis; Photometric redshifts; Physical machines; Pipeline; Platform; Platforms for big data process; Preprocessing; Radio astronomy; Radio telescope; Reionization; Ring buffers; Routing model; SKA; Source extraction; Spark; Square kilometer array; Statistical challenges; Stream processing; Telescope
438 p. · 19x23.3 cm · Paperback
Description
/li>Contents
/li>Readership
/li>Biography
/li>Comment
/li>
Big Data in Radio Astronomy: Scientific Data Processing for Advanced Radio Telescopes provides the latest research developments in big data methods and techniques for radio astronomy. Providing examples from such projects as the Square Kilometer Array (SKA), the world?s largest radio telescope that generates over an Exabyte of data every day, the book offers solutions for coping with the challenges and opportunities presented by the exponential growth of astronomical data. Presenting state-of-the-art results and research, this book is a timely reference for both practitioners and researchers working in radio astronomy, as well as students looking for a basic understanding of big data in astronomy.
Part A: Fundamentals
Chapter 1: Introduction of Radio Astronomy
Chapter 2: Fundamentals of Big Data in Radio Astronomy
Part B: Big Data Processing
Chapter 3: Pre-processing Pipeline on FPGA
Chapter 4: Real-time stream processing in radio astronomy
Chapter 5: Digitization, Channelization and Packeting
Chapter 6: Processing Data of Correlation on GPU
Chapter 7: Data Calibration for single dish radio telescope
Chapter 8: Imaging Algorithm Optimization for Scale-out Processing
Part C: Computing Technologies
Chapter 9: Execution Framework Technology
Chapter 10: Application Design For Execution Framework
Chapter 11: Heterogeneous Computing Platform for Backend Computing Tasks
Chapter 12: High Performance Computing for Astronomical Big Data
Chapter 13: Spark and Dask Performance Analysis Based on ARL Image Library
Chapter 14: Applications of Artificial Intelligence in Astrnomical Big Data
Part D: Future Developments
Chapter 15: Mapping the Universe with 21cm Observations
Tian Huang is Research Associate of the Astrophysics Group, Cavendish Lab, University of Cambridge. He takes part in multiple radio telescope array projects and mainly focuses on data preprocessing and quality metrics. In March 2016, he graduated from the School of Microelectronics at Shanghai Jiao Tong University, where he completed his PhD thesis. His main research interest is Data Mining for time series, including time series big data indexing, anomaly detecting, and computer architecture for time series data mining and statistical models for time series data. He has published 9 SCI journal and 18 EI conference papers. He has rich experience on software and hardware co-designing.
Yongxin Zhu is a full Professor at Shanghai Advanced Research Institute, Chinese Academy of Sciences (CAS). He is also an Adjunct Professor with the School of Microelectronics at the Shanghai Jiao Tong University (SJTU). He is currently the technical leader of Chinese Consortium of Science Da
- Bridges the gap between radio astronomy and computer science
- Includes coverage of the observation lifecycle as well as data collection, processing and analysis
- Presents state-of-the-art research and techniques in big data related to radio astronomy
- Utilizes real-world examples, such as Square Kilometer Array (SKA) and Five-hundred-meter Aperture Spherical radio Telescope (FAST)