Description
Data Analytics for Engineering and Construction Project Risk Management, 1st ed. 2020
Risk, Systems and Decisions Series
Language: English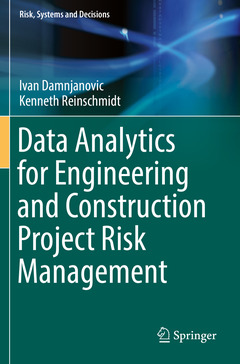
Subjects for Data Analytics for Engineering and Construction Project...:
Publication date: 07-2020
Support: Print on demand
Publication date: 06-2019
379 p. · 15.5x23.5 cm · Hardback
Description
/li>Contents
/li>Biography
/li>Comment
/li>
This book provides a step-by-step guidance on how to implement analytical methods in project risk management. The text focuses on engineering design and construction projects and as such is suitable for graduate students in engineering, construction, or project management, as well as practitioners aiming to develop, improve, and/or simplify corporate project management processes.
The book places emphasis on building data-driven models for additive-incremental risks, where data can be collected on project sites, assembled from queries of corporate databases, and/or generated using procedures for eliciting experts? judgments. While the presented models are mathematically inspired, they are nothing beyond what an engineering graduate is expected to know: some algebra, a little calculus, a little statistics, and, especially, undergraduate-level understanding of the probability theory.
The book is organized in three parts and fourteen chapters. In Part I theauthors provide the general introduction to risk and uncertainty analysis applied to engineering construction projects. The basic formulations and the methods for risk assessment used during project planning phase are discussed in Part II, while in Part III the authors present the methods for monitoring and (re)assessment of risks during project execution.
Kenneth F. Reinschmidt was a Professor Emeritus at Texas A&M University, a member of the National Academy of Engineering, and a fellow of the American Association for the Advancement of Science. During his long lasting career Dr Reinschmidt has held many positions in academia and industry including being a professor at the Massachusetts Institute of Technology, Senior Vice President of Stone & Webster, and the President and Chief Executive Officer of Stone & Webster Advanced Systems Development Services. He has pioneered many data-centred project management tools and methods including knowledge-based engineering analysis, computer-aided engineering design, and expert systems for manufacturing and production scheduling. Dr Reinschmidt served as a chairman or a member of many congressionally-mandated studies that investigated project management practices across different federal agencies. At Texas A&M University Dr. Reinschmidt used to teach project risk management and construction improvement methods.
Ivan D. Damnjanovic is an Associate Professor, J.L. “Corky” Frank/Marathon Ashland Petroleum LLC Faculty Fellow, and the Director of Engineering Project Management program at Texas A&M University. He is also the Founder of Riskopedia Analytics consultancy. Dr. Damnjanovic specializes in qualitative and quantitative methods for assessment and management of engineering and project risks. He has an extensive experience in risk and safety analysis applied to projects from different industry segments including transportation infrastructure, oil&gas, and technology development. Dr. Damnjanovic has been a lead investigator on more than 20 state and federally funded studies, and the author of more than 50 peer reviewed journal publications and reports. He has served as a member of a number of committees that looked into industry-wide applications of risk analysis. At Texas A&M University Dr. Damnjanovic teaches project risk management and project finan