Description
Analyzing Network Data in Biology and Medicine
An Interdisciplinary Textbook for Biological, Medical and Computational Scientists
Coordinator: Pržulj Nataša
Introduces biological concepts and biotechnologies producing the data, graph and network theory, cluster analysis and machine learning, using real-world biological and medical examples.
Language: English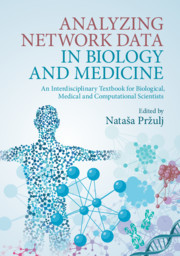
Subject for Analyzing Network Data in Biology and Medicine:
Analyzing Network Data in Biology and Medicine
Publication date: 03-2019
672 p. · Hardback
Publication date: 03-2019
672 p. · Hardback
Analyzing Network Data in Biology and Medicine
Publication date: 03-2019
643 p. · 17.5x24.7 cm · Paperback
Publication date: 03-2019
643 p. · 17.5x24.7 cm · Paperback
Description
/li>Contents
/li>Biography
/li>
The increased and widespread availability of large network data resources in recent years has resulted in a growing need for effective methods for their analysis. The challenge is to detect patterns that provide a better understanding of the data. However, this is not a straightforward task because of the size of the data sets and the computer power required for the analysis. The solution is to devise methods for approximately answering the questions posed, and these methods will vary depending on the data sets under scrutiny. This cutting-edge text introduces biological concepts and biotechnologies producing the data, graph and network theory, cluster analysis and machine learning, before discussing the thought processes and creativity involved in the analysis of large-scale biological and medical data sets, using a wide range of real-life examples. Bringing together leading experts, this text provides an ideal introduction to and insight into the interdisciplinary field of network data analysis in biomedicine.
1. From genetic data to medicine: from DNA samples to disease risk prediction in personalized genetic tests Luis Leal, Rok Košir and Nataša Pržulj; 2. Epigenetic data and disease Rodrigo González-Barrios, Marisol Salgado-Albarrán, Nicolás Alcaraz, Cristian Arriaga-Canon, Lissania Guerra-Calderas, Laura Contreras-Espinoza and Ernesto Soto-Reyes; 3. Introduction to graph and network theory Thomas Gaudelet and Nataša Pržulj; 4. Protein-protein interaction data, their quality, and major public databases Anne-Christin Hauschild, Chiara Pastrello, Max Kotlyar and Igor Jurisica; 5. Graphlets in network science and computational biology Khalique Newaz and Tijana Milenković; 6. Cluster analysis Richard Röttger; 7. Machine learning for data integration in cancer precision medicine: matrix factorization approaches Noël Malod-Dognin, Sam Windels and Nataša Pržulj; 8. Machine learning for biomarker discovery: significant pattern mining F. Llinares-Lopez and K. Borgwardt; 9. Network alignment Noël Malod-Dogning and Nataša Pržulj; 10. Network medicine Pisanu Buphamalai, Michael Caldera, Felix Müller and Jörg Menche; 11. Elucidating genotype-to-phenotype relationships via analyzes of human tissue interactomes Idan Hekselman, Moran Sharon, Omer Basha and Esti Yeger-Lotem; 12. Network neuroscience Alberto Cacciola, Alessandro Muscoloni and Carlo Vittorio Cannistraci; 13. Cytoscape: tool for analyzing and visualizing network data John H. Morris; 14. Analysis of the signatures of cancer stem cells in malignant tumours using protein interactomes and STRING database Krešimir Pavelić, Marko Klobučar, Dolores Kuzelj, Nataša Pržulj and Sandra Kraljević Pavelić.
Nataša Pržulj is Professor of Biomedical Data Science at University College London and an ICREA Research Professor at Barcelona Supercomputing Center. She has been an elected academician of The Academy of Europe, Academia Europaea, since 2017 and is a Fellow of the British Computer Society (BCS). She is recognized for designing methods to mine large real-world molecular network datasets and for extending and using machine learning methods for the integration of heterogeneous biomedical and molecular data, applied to advancing biological and medical knowledge. She received two prestigious European Research Council (ERC) research grants, Starting (2012–17) and Consolidator (2018–23), as well as USA National Science Foundation (NSF) grants, among others. She is a recipient of the BCS Roger Needham Award for 2014.
© 2024 LAVOISIER S.A.S.