Description
Multivariable Predictive Control
Applications in Industry
Author: Lahiri Sandip K.
Language: English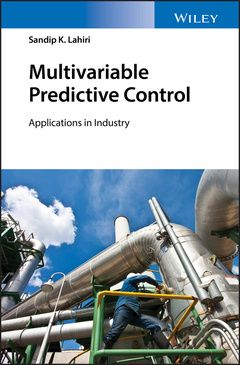
Subject for Multivariable Predictive Control:
Keywords
multivariate predictive controls; mpc; multivariate predictive control models; multivariate predictive control applications; multivariate predictive control software; mpc models; mpc applications; mpc software; mpc application examples; mpc application case studies; mpc failure; avoiding mpc failure; monitoring mpc performance; maintaining mpc applications; implementing mpc applications; monitoring mpc applications; mpc application guide for plant process engineers; mpc application guide for process control engineers; chemical process technology; petrochemical process technology; mpc software reviews; mpc systems safeguards; mpc application cost-benefit analysis; mpc project case studies; mpc application vendors; help with mpc applications; mpc advantages; maximising mpc systems benefits; model predictive controls; functional design of mpc controller
304 p. · 17x24.6 cm · Hardback
Description
/li>Contents
/li>Biography
/li>
A guide to all practical aspects of building, implementing, managing, and maintaining MPC applications in industrial plants
Multivariable Predictive Control: Applications in Industry provides engineers with a thorough understanding of all practical aspects of multivariate predictive control (MPC) applications, as well as expert guidance on how to derive maximum benefit from those systems. Short on theory and long on step-by-step information, it covers everything plant process engineers and control engineers need to know about building, deploying, and managing MPC applications in their companies.
MPC has more than proven itself to be one the most important tools for optimising plant operations on an ongoing basis. Companies, worldwide, across a range of industries are successfully using MPC systems to optimise materials and utility consumption, reduce waste, minimise pollution, and maximise production. Unfortunately, due in part to the lack of practical references, plant engineers are often at a loss as to how to manage and maintain MPC systems once the applications have been installed and the consultants and vendors? reps have left the plant. Written by a chemical engineer with two decades of experience in operations and technical services at petrochemical companies, this book fills that regrettable gap in the professional literature.
- Provides a cost-benefit analysis of typical MPC projects and reviews commercially available MPC software packages
- Details software implementation steps, as well as techniques for successfully evaluating and monitoring software performance once it has been installed
- Features case studies and real-world examples from industries, worldwide, illustrating the advantages and common pitfalls of MPC systems
- Describes MPC application failures in an array of companies, exposes the root causes of those failures, and offers proven safeguards and corrective measures for avoiding similar failures
Multivariable Predictive Control: Applications in Industry is an indispensable resource for plant process engineers and control engineers working in chemical plants, petrochemical companies, and oil refineries in which MPC systems already are operational, or where MPC implementations are being considering.
Figure List xix
Table List xxi
Preface xxiii
1 Introduction of Model Predictive Control 1
1.1 Purpose of Process Control in Chemical Process Industries (CPI) 1
1.2 Shortcomings of Simple Regulatory PID Control 2
1.3 What Is Multivariable Model Predictive Control? 3
1.4 Why Is a Multivariable Model Predictive Optimizing Controller Necessary? 4
1.5 Relevance of Multivariable Predictive Control (MPC) in Chemical Process Industry in Today’s Business Environment 6
1.6 Position of MPC in Control Hierarchy 6
1.6.1 Regulatory PID Control Layer 6
1.6.2 Advance Regulatory Control (ARC) Layer 8
1.6.3 Multivariable Model‐Based Control 8
1.6.4 Economic Optimization Layer 8
1.6.4.1 First Layer of Optimization 8
1.6.4.2 Second Layer of Optimization 9
1.6.4.3 Third Layer of Optimization 9
1.7 Advantage of Implementing MPC 10
1.8 How Does MPC Extract Benefit? 13
1.8.1 MPC Inherent Stabilization Effect 13
1.8.2 Process Interactions 14
1.8.3 Multiple Constraints 15
1.8.4 Intangible Benefits of MPC 17
1.9 Application of MPC in Oil Refinery, Petrochemical, Fertilizer, and Chemical Plants, and Related Benefits 17
2 Theoretical Base of MPC 23
2.1 Why MPC? 23
2.2 Variables Used in MPC 25
2.2.1 Manipulated Variables (MVs) 25
2.2.2 Controlled Variables (CVs) 25
2.2.3 Disturbance Variables (DVs) 25
2.3 Features of MPC 26
2.3.1 MPC Is a Multivariable Controller 26
2.3.2 MPC Is a Model Predictive Controller 26
2.3.3 MPC Is a Constrained Controller 26
2.3.4 MPC Is an Optimizing Controller 27
2.3.5 MPC Is a Rigorous Controller 27
2.4 Brief Introduction to Model Predictive Control Techniques 27
2.4.1 Simplified Dynamic Control Strategy of MPC 28
2.4.2 Step 1: Read Process Input and Output 29
2.4.3 Step 2: Prediction of CVs 30
2.4.3.1 Building Dynamic Process Model 30
2.4.3.2 How MPC Predicts the Future 32
2.4.4 Step 3: Model Reconciliation 33
2.4.5 Step 4: Determine the Size of the Control Process 34
2.4.6 Step 5: Removal of Ill‐Conditioned Problems 34
2.4.7 Step 6: Optimum Steady‐State Targets 35
2.4.8 Step 7: Develop Detailed Plan of MV Movement 36
3 Historical Development of Different MPC Technology 43
3.1 History of MPC Technology 43
3.1.1 Pre‐Era 43
3.1.1.1 Developer 43
3.1.1.2 Motivation 44
3.1.1.3 Limitations 44
3.1.2 First Generation of MPC (1970–1980) 44
3.1.2.1 Characteristics of First‐Generation MPC Technology 44
3.1.2.2 IDCOM Algorithm and Its Features 45
3.1.2.3 DMC Algorithm and Its Features 46
3.1.3 Second‐Generation MPC (1980–1985) 46
3.1.4 Third‐Generation MPC (1985–1990) 47
3.1.4.1 Distinguishing Features of Third‐Generation MPC Algorithm 48
3.1.4.2 Distinguishing Features of the IDCOM‐M Algorithm 49
3.1.4.3 Evolution of SMOC 50
3.1.4.4 Distinctive Features of SMOC 50
3.1.5 Fourth‐Generation MPC (1990–2000) 50
3.1.5.1 Distinctive Features of Fourth‐Generation MPC 51
3.1.6 Fifth‐Generation MPC (2000–2015) 51
3.2 Points to Consider While Selecting an MPC 52
4 MPC Implementation Steps 55
4.1 Implementing a MPC Controller 55
4.1.1 Step 1: Preliminary Cost–Benefit Analysis 55
4.1.2 Step 2: Assessment of Base Control Loops 55
4.1.3 Step 3: Functional Design of Controller 56
4.1.4 Step 4: Conduct the Preliminary Plant Test (Pre‐Stepping) 57
4.1.5 Step 5: Conduct the Plant Step Test 57
4.1.6 Step 6: Identify a Process Model 57
4.1.7 Step 7: Generate Online Soft Sensors or Virtual Sensors 58
4.1.8 Step 8: Perform Offline Controller Simulation/Tuning 58
4.1.9 Step 9: Commission the Online Controller 58
4.1.10 Step 10: Online MPC Controller Tuning 59
4.1.11 Step 11: Hold Formal Operator Training 59
4.1.12 Step 12: Performance Monitoring of MPC Controller 59
4.1.13 Step 13: Maintain the MPC Controller 60
4.2 Summary of Steps Involved in MPC Projects with Vendor 60
5 Cost–Benefit Analysis of MPC before Implementation 63
5.1 Purpose of Cost–Benefit Analysis of MPC before Implementation 63
5.2 Overview of Cost–Benefit Analysis Procedure 64
5.3 Detailed Benefit Estimation Procedures 65
5.3.1 Initial Screening for Suitability of Process to Implement MPC 65
5.3.2 Process Analysis and Economics Analysis 66
5.3.3 Understand the Constraints 67
5.3.4 Identify Qualitatively Potential Area of Opportunities 67
5.3.4.1 Example 1: Air Separation Plant 68
5.3.4.2 Example 2: Distillation Columns 69
5.3.5 Collect All Relevant Plant and Economic Data (Trends, Records) 69
5.3.6 Calculate the Standard Deviation and Define the Limit 69
5.3.7 Estimate the Stabilizing Effect of MPC and Shift in the Average 70
5.3.7.1 Benefit Estimation: When the Constraint Is Known 71
5.3.7.2 Benefit Estimation: When the Constraint Is Not Well Known or Changing 72
5.3.8 Estimate Change in Key Performance Parameters Such as Yield, Throughput, and Energy Consumption 72
5.3.8.1 Example: Ethylene Oxide Reactor 72
5.3.9 Identify How This Effect Translates to Plant Profit Margin 73
5.3.10 Estimate the Economic Value of the Effect 73
5.4 Case Studies 73
5.4.1 Case Study 1 73
5.4.1.1 Benefit Estimation Procedure 73
5.4.2 Case Study 2 74
5.4.2.1 Benefit Estimation Procedure 74
6 Assessment of Regulatory Base Control Layer in Plants 77
6.1 Failure Mode of Control Loops and Their Remedies 77
6.2 Control Valve Problems 77
6.2.1 Improper Valve Sizing 78
6.2.1.1 How to Detect a Particular Control Valve Sizing Problem 78
6.2.2 Valve Stiction 79
6.2.2.1 What Is Control Valve Stiction? 79
6.2.2.2 How to Detect Control Valve Stiction Online 80
6.2.2.3 Combating Stiction 80
6.2.2.4 Techniques for Combating Stiction Online 80
6.2.3 Valve Hysteresis and Backlash 81
6.3 Sensor Problems 82
6.3.1 Noisy 82
6.3.2 Flatlining 82
6.3.3 Scale/Range 82
6.3.4 Calibration 82
6.3.5 Overfiltered 83
6.4 Controller Problems 83
6.4.1 Poor Tuning and Lack of Maintenance 83
6.4.2 Poor or Missing Feedforward Compensation 83
6.4.3 Inappropriate Control Structure 84
6.5 Process‐Related Problems 84
6.5.1 Problems of Variable Gain 84
6.5.2 Oscillations 84
6.5.2.1 Variable Valve Gain 85
6.5.2.2 Variable Process Gain 85
6.6 Human Factor 85
6.7 Control Performance Assessment/Monitoring 86
6.7.1 Available Software for Control Performance Monitoring 86
6.7.2 Basic Assessment Procedure 87
6.8 Commonly Used Control System Performance KPIs 87
6.8.1 Traditional Indices 88
6.8.1.1 Peak Overshoot Ratio (POR) 88
6.8.1.2 Decay Rate 88
6.8.1.3 Peak Time and Rise Time 88
6.8.1.4 Settling Time 88
6.8.1.5 Integral of Error Indexes 88
6.8.2 Simple Statistical Indices 88
6.8.2.1 Mean of Control Error (%) 89
6.8.2.2 Standard Deviation of Control Error (%) 89
6.8.2.3 Standard Variation of Control Error (%) 89
6.8.2.4 Standard Deviation of Controller Output (%) 89
6.8.2.5 Skewness of Control Error 89
6.8.2.6 Kurtosis of Control Error 89
6.8.2.7 Ratio of Standard of Control Error and Controller Output 89
6.8.2.8 Maximum Bicoherence 90
6.8.3 Business/Operational Metrics 90
6.8.3.1 Loop Health 90
6.8.3.2 Service Factor 90
6.8.3.3 Key Performance Indicators 90
6.8.3.4 Operational Performance Efficiency Factor 90
6.8.3.5 Overall Loop Performance Index 90
6.8.3.6 Controller Output Changes in Manual 90
6.8.3.7 Mode Changes 90
6.8.3.8 Totalized Valve Reversals and Valve Travel 90
6.8.3.9 Process Model Parameters 90
6.8.4 Advanced Indices 90
6.8.4.1 Harris Index 91
6.8.4.2 Nonlinearity Index 91
6.8.4.3 Oscillation‐Detection Indices 91
6.8.4.4 Disturbance Detection Indices 92
6.8.4.5 Autocorrelation Indices 92
6.9 Tuning for PID Controllers 92
6.9.1 Complications with Tuning PID Controllers 93
6.9.2 Loop Retuning 93
6.9.3 Classical Controller Tuning Algorithms 94
6.9.3.1 Controller Tuning Methods 94
6.9.3.2 Ziegler‐Nichols Tuning Method 94
6.9.3.3 Dahlin (Lambda) Tuning Method 94
6.9.4 Manual Controller Tuning Methods in Absence of Any Software 95
6.9.4.1 Pre‐Tuning 95
6.9.4.2 Bring in Baseline Parameters 97
6.9.4.3 Some Like It Simple 97
6.9.4.4 Tuning Cascade Control 98
7 Functional Design of MPC Controllers 101
7.1 What Is Functional Design? 101
7.2 Steps in Functional Design 102
7.2.1 Step 1: Define Process Control Objectives 102
7.2.1.1 Economic Objectives 102
7.2.1.2 Operating Objectives 103
7.2.1.3 Control Objectives 104
7.2.2 Step 2: Identify Process Constraints 104
7.2.2.1 Process Limitations 104
7.2.2.2 Safety Limitations 104
7.2.2.3 Process Instrument Limitations 105
7.2.2.4 Raw Material and Utility Supply Limitation 105
7.2.2.5 Product Limitations 105
7.2.3 Step 3: Define Controller Scope 105
7.2.4 Step 4: Select the Variables 106
7.2.4.1 Economics of the Unit 106
7.2.4.2 Constraints of the Unit 107
7.2.4.3 Control of the Unit 107
7.2.4.4 Manipulated Variables (MVs) 107
7.2.4.5 Controlled Variables (CVs) 107
7.2.4.6 Disturbance Variables (DVs) 108
7.2.4.7 Practical Guidelines for Variable Selections 108
7.2.5 Step 5: Rectify Regulatory Control Issues 109
7.2.5.1 Practical Guidelines for Changing Regulatory Controller Strategy 109
7.2.6 Step 6: Explore the Scope of Inclusions of Inferential Calculations 110
7.2.7 Step 7: Evaluate Potential Optimization Opportunity 110
7.2.7.1 Practical Guidelines for Finding out Optimization Opportunities 111
7.2.8 Step 8: Define LP or QP Objective Function 111
7.2.8.1 CDU Example 112
8 Preliminary Process Test and Step Test 113
8.1 Pre‐Stepping, or Preliminary Process Test 113
8.1.1 What Is Pre‐Stepping? 113
8.1.2 Objective of Pre‐Stepping 113
8.1.3 Prerequisites of Pre‐Stepping 113
8.1.4 Pre‐Stepping 114
8.2 Step Testing 115
8.2.1 What Is a Step Test? 115
8.2.2 What Is the Purpose of a Step Test? 115
8.2.3 Details of Step Testing 116
8.2.3.1 Administrative Aspects 116
8.2.3.2 Technical Aspects 116
8.2.4 Different Step‐Testing Method 117
8.2.4.1 Manual Step Testing 117
8.2.4.2 PRBS (Pseudo Random Binary Sequence) 117
8.2.4.3 General Guidelines of PRBS Test 117
8.2.5 Difference between Normal Step Testing and PRBS Testing 118
8.2.6 Which One to Choose? 118
8.2.7 Dos and Don’ts of Step Testing 118
8.3 Development of Step‐Testing Methodology over the Years 120
9 Model Building and System Identification 123
9.1 Introduction to Model Building 123
9.2 Key Issues in Model Identifications 124
9.2.1 Identification Test 124
9.2.2 Model Structure and Parameter Estimation 125
9.2.3 Order Selection 126
9.2.4 Model Validation 127
9.3 The Basic Steps of System Identification 127
9.3.1 Step 0: Experimental Design and Execution 128
9.3.2 Step 1: Plan the Case that Needs to Be Modeled 130
9.3.2.1 Action 1 130
9.3.2.2 Action 2 130
9.3.3 Step 2: Identify Good Slices of Data 130
9.3.3.1 Looking at the Data 131
9.3.4 Step 3: Pre‐Processing of Data 131
9.3.5 Step 4: Identification of Model Curve 132
9.3.5.1 Hybrid Approach to System Identification 132
9.3.5.2 Direct Modeling Approach of System Identification 133
9.3.5.3 Subspace Identification 134
9.3.5.4 Detailed Steps of Implementations 135
9.3.6 Step 5: Select Final Model 136
9.4 Model Structures 137
9.4.1 FIR Models 138
9.4.1.1 FIR Structures 138
9.4.2 Prediction Error Models (PEM Models) 139
9.4.2.1 PEM Structures 139
9.4.3 Model for Order and Variance Reduction 140
9.4.3.1 ARX Parametric Models (Discrete Time) 140
9.4.3.2 Output Error Models (Discrete Time) 140
9.4.3.3 Laplace Domain Parametric Models 141
9.4.3.4 Final Model Form 141
9.4.4 State‐Space Models 141
9.4.5 How to Know Which Structure and Method to Use 142
9.5 Common Features of Commercial Identification Packages 142
10 Soft Sensors 145
10.1 What Is a Soft Sensor? 145
10.2 Why Soft Sensors Are Necessary 145
10.2.1 Process Monitoring and Process Fault Detection 146
10.2.2 Sensor Fault Detection and Reconstruction 146
10.2.3 Use of Soft Sensors in MPC Application 146
10.3 Types of Soft Sensors 147
10.3.1 First Principle‐Based Soft Sensors 147
10.3.1.1 Advantages 147
10.3.1.2 Disadvantages 147
10.3.2 Data‐Driven Soft Sensors 148
10.3.2.1 Advantages 148
10.3.2.2 Disadvantages 148
10.3.3 Gray Model‐Based Soft Sensors 148
10.3.3.1 Advantages 149
10.3.4 Hybrid Model‐Based Soft Sensors 149
10.3.4.1 Advantages 149
10.4 Soft Sensors Development Methodology 149
10.4.1 Data Collection and Data Inspection 149
10.4.2 Data Preprocessing and Data Conditioning 150
10.4.2.1 Outlier Detection and Replacement 151
10.4.2.2 Univariate Approach to Detect Outliers 151
10.4.2.3 Multivariate Approach to Detect Outliers (Lin 2007) 151
10.4.2.4 Handling of Missing Data 152
10.4.3 Selection of Relevant Input Output Variables 153
10.4.4 Data Alignment 153
10.4.5 Model Selection, Training, and Validation (Kadlec 2009; Lin 2007) 153
10.4.6 Analyze Process Dynamics 154
10.4.7 Deployment and Maintenance 155
10.5 Data‐Driven Methods for Soft Sensing 156
10.5.1 Principle Component Analysis 156
10.5.1.1 The Basics of PCA 156
10.5.1.2 Why Do We Need to Rotate the Data? 156
10.5.1.3 How Do We Generate Principal Components? 156
10.5.1.4 Steps to Calculating Principal Components 157
10.5.2 Partial Least Squares 157
10.5.3 Artificial Neural Networks 158
10.5.3.1 Network Architecture 159
10.5.3.2 Back Propagation Algorithm (BPA) 159
10.5.4 Neuro‐Fuzzy Systems 160
10.5.5 Support Vector Machines 161
10.5.5.1 Support Vector Regression–Based Modeling 161
10.6 Open Issues and Future Steps of Soft Sensor Development 162
10.6.1 Large Effort Required for Preprocessing of Industrial Data 162
10.6.2 Which Modeling Method to Choose? 163
10.6.3 Agreement of the Developed Model with Physics of the Process 163
10.6.4 Performance Deterioration of Developed Soft Sensor Model 163
11 Offline Simulation 167
11.1 What Is Offline Simulation? 167
11.2 Purpose of Offline Simulation 167
11.3 Main Task of Offline Simulation 168
11.4 Understanding Different Tuning Parameters of Offline Simulations 168
11.4.1 Tuning Parameters for CVs 169
11.4.1.1 Methods for Handling of Infeasibility 170
11.4.1.2 Priority Ranking of CVs 170
11.4.1.3 cv Give‐Up 170
11.4.1.4 cv Error Weight 170
11.4.2 Tuning Parameters for MVs 171
11.4.2.1 mv Maximum Movement Limits or Rate‐of‐Change Limits 171
11.4.2.2 Movement Weights 171
11.4.3 Tuning Parameters for Optimizer 172
11.4.3.1 Economic Optimization 172
11.4.3.2 General Form of Objective Function 173
11.4.3.3 Weighting Coefficients 173
11.4.3.4 Setting Linear Objective Coefficients 173
11.4.3.5 Optimization Horizon and Optimization Speed Factor 174
11.4.3.6 Optimization Speed Factor 174
11.4.3.7 mv Optimization Priority 174
11.4.4 Soft Limits 175
11.4.4.1 How Soft Limits Work 175
11.4.4.2 cv Soft Limits 175
11.4.4.3 mv Soft Limits 176
11.5 Different Steps to Build and Activate Simulator in an Offline PC 176
11.6 Example of Tests Carried out in Simulator 177
11.6.1 Control and Optimization Objectives 177
11.6.1.1 Test 1 178
11.6.1.2 Test 2 179
11.6.1.3 Test 3 179
11.6.1.4 Test 4 180
11.6.1.5 Test 5 180
11.6.1.6 Test 6 180
11.6.1.7 Others Tests 181
11.7 Guidelines for Choosing Tuning Parameters 181
11.7.1 Guidelines for Choosing Initial Values 181
11.7.2 How to Select Maximum Move Size and MV Movement Weights During Simulation Study 182
12 Online Deployment of MPC Application in Real Plants 183
12.1 What Is Online Deployment (Controller Commissioning)? 183
12.2 Steps for Controller Commissioning 183
12.2.1 Set up the Controller Configuration and Final Review of the Model 183
12.2.2 Build the Controller 184
12.2.3 Load Operator Station on PC Near the Panel Operator 184
12.2.4 Take MPC Controller in Line with Prediction Mode 186
12.2.5 Put the MPC Controller in Close Loop with One CV at a Time 187
12.2.6 Observe MPC Controller Performance 187
12.2.7 Put Optimizer in Line and Observe Optimizer Performance 189
12.2.8 Evaluate Overall Controller Performance 189
12.2.9 Perform Online Tuning and Troubleshooting 190
12.2.10 Train Operators and Engineers on Online Platform 190
12.2.11 Document MPC Features 190
12.2.12 Maintain the MPC Controller 191
13 Online Controller Tuning 193
13.1 What Is Online MPC Controller Tuning? 193
13.2 Basics of Online Tuning 193
13.2.1 Key Checkout Regarding Controller Performance 193
13.2.2 Steps to Troubleshoot the Problem 194
13.3 Guidelines to Choose Different Tuning Parameters 195
14 Why Do Some MPC Applications Fail? 199
14.1 What Went Wrong? 199
14.2 Failure to Build Efficient MPC Application 201
14.2.1 Historical Perspective 201
14.2.2 Capability of MPC Software to Capture Benefits 202
14.2.3 Expertise of Implementation Team 202
14.2.3.1 MPC Vendor Limitations 203
14.2.3.2 Client Limitations 204
14.2.4 Reliability of APC Project Methodology 204
14.3 Contributing Failure Factors of Postimplementation MPC Application 205
14.3.1 Technical Failure Factors 206
14.3.1.1 Lack of Performance Monitoring of MPC Application 206
14.3.1.2 Unresolved Basic Control Problems 206
14.3.1.3 Poor Tuning and Degraded Model Quality 207
14.3.1.4 Problems Related to Controller Design 207
14.3.1.5 Significant Process Modifications and Enhancement 207
14.3.2 Nontechnical Failure Factors 208
14.3.2.1 Lack of Properly Trained Personnel 208
14.3.2.2 Lack of Standards and Guidelines to MPC Support Personnel 208
14.3.2.3 Lack of Organizational Collaboration and Alignment 208
14.3.2.4 Poor Management of Control System 209
14.4 Strategies to Avoid MPC Failures 210
14.4.1 Technical Solutions 211
14.4.1.1 Development of Online Performance Monitoring of APC Applications 211
14.4.1.2 Improvement of Base Control Layer 212
14.4.1.3 Tuning Basic Controls 212
14.4.1.4 Control Performance Monitoring Software 213
14.4.2 Management Solutions 214
14.4.2.1 Training of MPC Console Operators 214
14.4.2.2 Training of MPC Control Engineers 215
14.4.2.3 Development of Corporate MPC Standards and Guidelines 216
14.4.2.4 Central Engineering Support Organization for MPC 217
14.4.3 Outsourcing Solutions 219
15 MPC Performance Monitoring 221
15.1 Why Performance Assessment of MPC Application Is Necessary 221
15.2 Types of Performance Assessment 222
15.2.1 Control Performance 222
15.2.2 Optimization Performance 222
15.2.3 Economic Performance 222
15.2.4 Intangible Performance 222
15.3 Benefit Measurement after MPC Implementation 222
15.4 Parameters to Be Monitored for MPC Performance Evaluation 223
15.4.1 Service Factors 224
15.4.2 KPI for Financial Criteria 224
15.4.3 KPI for Standard Deviation of Key Process Variable 225
15.4.3.1 Safety Parameters 225
15.4.3.2 Quality Giveaway Parameters 225
15.4.3.3 Economic Parameters 225
15.4.4 KPI for Constraint Activity 226
15.4.5 KPI for Constraint Violation 226
15.4.6 KPI for Inferential Model Monitoring 226
15.4.7 Model Quality 226
15.4.8 Limit Change Frequencies for CV/MVs 227
15.4.9 Active MV Limit 227
15.4.10 Long‐Term Performance Monitoring of MPC 227
15.5 KPIs to Troubleshoot Poor Performance of Multivariable Controls 228
15.5.1 Supporting KPIs for Low Service Factor 228
15.5.2 KPIs to Troubleshoot Cycling 229
15.5.3 KPIs for Oscillation Detection 230
15.5.4 KPIs for Regulatory Control Issues 230
15.5.5 KPIs for Measuring Operator Actions 231
15.5.6 KPIs for Measuring Process Changes and Disturbances 231
15.6 Exploitation of Constraints Handling and Maximization of MPC Benefit 231
16 Commercial MPC Vendors and Applications 235
16.1 Basic Modules and Components of Commercial MPC Software 235
16.1.1 Basic MPC Package 235
16.1.2 Data Collection Module 236
16.1.3 MPC Online Controller 236
16.1.4 Operator/ Engineer Station 237
16.1.5 System Identification Module 237
16.1.5.1 Different Modeling Options 239
16.1.5.2 Reporting and Documentation Function 239
16.1.5.3 Data Analysis and Pre‐Processing 239
16.1.6 PC‐Based Offline Simulation Package 240
16.1.7 Control Performance Monitoring and Diagnostics Software 240
16.1.7.1 Control Performance Monitoring 240
16.1.7.2 Basic Features of Performance Monitoring and Diagnostics Software 240
16.1.7.3 Performance and Benefits Metrics 241
16.1.7.4 Offline Module 241
16.1.7.5 Online Package 241
16.1.7.6 Online Reports 241
16.1.8 Soft Sensor Module (Also Called Quality Estimator Module) 242
16.1.8.1 Soft Sensor Offline Package 242
16.1.8.2 Soft Sensor Online Package 243
16.1.8.3 Soft Sensor Module Simulation Tool 243
16.2 Major Commercial MPC Software 243
16.3 AspenTech and DMCplus 244
16.3.1 Brief History of Development 244
16.3.1.1 Enhancement of DMC Technology to QDMC Technology in 1983, Regarded as Second‐Generation of MPC Technology (1980–1985) 244
16.3.1.2 Introduction of AspenTech and Evolvement of Third‐Generation MPC Technology (1985–1990) 245
16.3.1.3 Appearance of DMCplus Product with Fourth‐Generation MPC Technology (1990–2000) 245
16.3.1.4 Improvement of DMCplus Technology for Quicker Implementation in Shop Floor, Regarded as Fifth‐Generation MPC (2000–2015) 245
16.3.2 DMCplus Product Package 246
16.3.2.1 Aspen DMCplus Desktop 246
16.3.2.2 Aspen DMCplus Online 246
16.3.2.3 DMCplus Models and Identification Package 247
16.3.2.4 Aspen IQ (Soft Sensor Software) 247
16.3.2.5 Aspen Watch: AspenTech MPC Monitoring and Diagnostic Software 247
16.3.3 Distinctive Features of DMCplus Software Package 248
16.3.3.1 Automating Best Practices in Process Unit Step Testing 248
16.3.3.2 Adaptive Modeling 248
16.3.3.3 New Innovation 249
16.3.3.4 Background Step Testing 250
16.4 RMPCT by Honeywell 251
16.4.1 Brief History of Development 251
16.4.2 Honeywell MPC Product Package and Its Special Features 251
16.4.3 Key Features and Functions of RMPCT 251
16.4.3.1 Special Feature to Handle Model Error 251
16.4.3.2 Coping with Model Error 252
16.4.3.3 Funnels 252
16.4.3.4 Range Control Algorithm 252
16.4.4 Product Value Optimization Capabilities 252
16.4.5 “One‐Knob” Tuning 253
16.5 SMOC–Shell Global Solution 253
16.5.1 Evolution of Advance Process Control in Shell 253
16.5.1.1 1975–1998: The Beginnings 253
16.5.1.2 1998–2008: Shell Global Solution and Partnering with Yokogawa Era 254
16.5.1.3 2008 Onward: Shell Returns to Its Own Application 254
16.5.2 Shell MPC Product Package and Its Special Features 255
16.5.2.1 Key Characteristics of SMOC 255
16.5.2.2 Applications 255
16.5.3 SMOC Integrated Software Modules 255
16.5.3.1 AIDA Pro Offline Modeling Package 256
16.5.3.2 md Pro 256
16.5.3.3 RQE Pro 256
16.5.3.4 SMOC Pro 257
16.5.4 SMOC Claim of Superior Distinctive Features 259
16.5.4.1 Integrated Dynamic Modeling Tools and Automatic Step Tests 259
16.5.4.2 State‐of‐the‐Art Online Commissioning Tools 259
16.5.4.3 Online Tuning 259
16.5.4.4 Advance Regulatory Controls 260
16.5.4.5 Features of New Product 260
16.6 Conclusion 261
Index 263
Sandip Kumar Lahiri, PhD, is a chemical engineer with more than twenty one years of experience in operations and technical services at leading petrochemical industries around the globe. His areas of expertise include simulation, process modelling, artificial intelligence and neural networks in process industry, APC, soft sensor, and slurry flow modelling.