Description
Improved Classification Rates for Localized Algorithms under Margin Conditions, 1st ed. 2020
Author: Blaschzyk Ingrid Karin
Language: English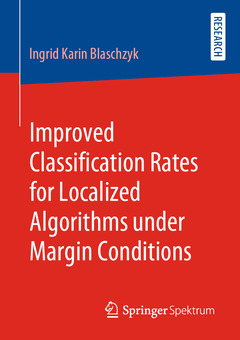
Subjects for Improved Classification Rates for Localized Algorithms...:
Approximative price 52.74 €
In Print (Delivery period: 15 days).
Add to cart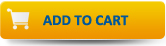
Publication date: 03-2020
126 p. · 14.8x21 cm · Paperback
126 p. · 14.8x21 cm · Paperback
Description
/li>Contents
/li>Biography
/li>Comment
/li>
Support vector machines (SVMs) are one of the most successful algorithms on small and medium-sized data sets, but on large-scale data sets their training and predictions become computationally infeasible. The author considers a spatially defined data chunking method for large-scale learning problems, leading to so-called localized SVMs, and implements an in-depth mathematical analysis with theoretical guarantees, which in particular include classification rates. The statistical analysis relies on a new and simple partitioning based technique and takes well-known margin conditions into account that describe the behavior of the data-generating distribution. It turns out that the rates outperform known rates of several other learning algorithms under suitable sets of assumptions. From a practical point of view, the author shows that a common training and validation procedure achieves the theoretical rates adaptively, that is, without knowing the margin parameters in advance.
Introduction to Statistical Learning Theory.- Histogram Rule: Oracle Inequality and Learning Rates.- Localized SVMs: Oracle Inequalities and Learning Rates.
Ingrid Karin Blaschzyk is a postdoctoral researcher in the Department of Mathematics at the University of Stuttgart, Germany.
Study in the field of natural sciences
Study in the field of statistical learning theory
© 2024 LAVOISIER S.A.S.
These books may interest you
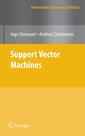
Support Vector Machines 163.51 €