Description
Missing Data Analysis in Practice
Chapman & Hall/CRC Interdisciplinary Statistics Series
Author: Raghunathan Trivellore
Language: English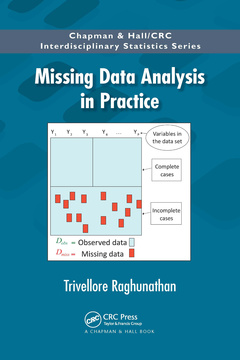
Subjects for Missing Data Analysis in Practice:
Keywords
MAR Mechanism; Multiple Imputation; Sensitivity Analysis; Missing Data Mechanism; Weighting methods; Missing Data; Ignorable missing data mechanisms; Inverse Probability Weighting; non-ignorable missing data mechanisms; Cumulative Distribution Function; ANOVA Method; Missing Values; Data Set; Longitudinal Analysis; Imputation Model; Regression Analysis; Post-stratified Estimator; Multiply Imputed Data Sets; Complete Case Analysis; Regression Model; Nonignorable Missing Data Mechanisms; Response Propensity; Imputed Values; Software Mouse; Multiple Imputation Estimate; Dummy Variable; Sequential Regression Approach; Scatter Plots; Synthetic Data Sets; Hot Deck; Post-stratification Weights; Hot Deck Method
Publication date: 12-2020
· 15.6x23.4 cm · Paperback
Publication date: 10-2015
· 15.6x23.4 cm · Hardback
Description
/li>Contents
/li>Readership
/li>Biography
/li>
Missing Data Analysis in Practice provides practical methods for analyzing missing data along with the heuristic reasoning for understanding the theoretical underpinnings. Drawing on his 25 years of experience researching, teaching, and consulting in quantitative areas, the author presents both frequentist and Bayesian perspectives. He describes easy-to-implement approaches, the underlying assumptions, and practical means for assessing these assumptions. Actual and simulated data sets illustrate important concepts, with the data sets and codes available online.
The book underscores the development of missing data methods and their adaptation to practical problems. It mainly focuses on the traditional missing data problem. The author also shows how to use the missing data framework in many other statistical problems, such as measurement error, finite population inference, disclosure limitation, combing information from multiple data sources, and causal inference.
Basic Concepts. Weighting Methods. Imputation. Multiple Imputation. Regression Analysis. Longitudinal Analysis with Missing Values. Nonignorable Missing Data Mechanisms. Other Applications. Other Topics. Bibliography. Index.
Trivellore Raghunathan is the director of the Survey Research Center in the Institute for Social Research and professor of biostatistics in the School of Public Health at the University of Michigan. He has published numerous papers in a range of statistical and public health journals. His research interests include applied regression analysis, linear models, design of experiments, sample survey methods, and Bayesian inference.
These books may interest you
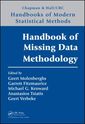
Handbook of Missing Data Methodology 123.78 €