Description
Sample Size Calculations for Clustered and Longitudinal Outcomes in Clinical Research
Chapman & Hall/CRC Biostatistics Series
Authors: Ahn Chul, Heo Moonseoung, Zhang Song
Language: English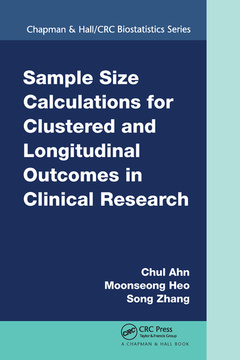
Subjects for Sample Size Calculations for Clustered and Longitudinal...:
Keywords
Sample Size Calculation; Cluster Randomization Trials; formulas; Sample Size Formula; required; Sample Size Estimate; estimate; Alternative Hypothesis H1; determination; Fixed Slope Model; Sample Size N3; hypothesis; Required Sample Size; randomization; Correlation Structure; trials; Sample Size Determination; treatment; Intracluster Correlation; groups; Missing Data; Mm Pattern; Difference Score Analysis; Repeated Measurement Outcomes; Arbitrary Correlation Structures; Non-inferiority Trial; Repeated Measurement Studies; Non-central Chi Square Distribution; Missing Patterns; Cluster Size; Stepped Wedge Design; Minimum Detectable Effect Sizes; Average Bioequivalence; Noninferiority Trials
57.55 €
In Print (Delivery period: 14 days).
Add to cart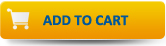
Publication date: 06-2020
· 15.6x23.4 cm · Paperback
107.92 €
In Print (Delivery period: 15 days).
Add to cart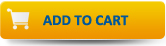
Publication date: 12-2014
· 15.6x23.4 cm · Hardback
Description
/li>Contents
/li>Readership
/li>Biography
/li>
Accurate sample size calculation ensures that clinical studies have adequate power to detect clinically meaningful effects. This results in the efficient use of resources and avoids exposing a disproportionate number of patients to experimental treatments caused by an overpowered study.
Sample Size Calculations for Clustered and Longitudinal Outcomes in Clinical Research explains how to determine sample size for studies with correlated outcomes, which are widely implemented in medical, epidemiological, and behavioral studies.
The book focuses on issues specific to the two types of correlated outcomes: longitudinal and clustered. For clustered studies, the authors provide sample size formulas that accommodate variable cluster sizes and within-cluster correlation. For longitudinal studies, they present sample size formulas to account for within-subject correlation among repeated measurements and various missing data patterns. For multiple levels of clustering, the level at which to perform randomization actually becomes a design parameter. The authors show how this can greatly impact trial administration, analysis, and sample size requirement.
Addressing the overarching theme of sample size determination for correlated outcomes, this book provides a useful resource for biostatisticians, clinical investigators, epidemiologists, and social scientists whose research involves trials with correlated outcomes. Each chapter is self-contained so readers can explore topics relevant to their research projects without having to refer to other chapters.
Sample Size Determination for Independent Outcomes. Sample Size Determination for Clustered Outcomes. Sample Size Determination for Repeated Measurement Outcomes Using Summary Statistics. Sample Size Determination for Correlated Outcome Measurements Using GEE. Sample Size Determination for Correlated Outcomes from Two-Level Randomized Clinical Trials. Sample Size Determination for Correlated Outcomes from Three Level Randomized Clinical Trials.
Chul Ahn, PhD, is a professor in the Department of Clinical Sciences and the cancer center associate director for biostatistics and bioinformatics in the Simmons Comprehensive Cancer Center at the University of Texas Southwestern Medical Center. He is also director of Biostatistics and Research Design for the NIH-sponsored Clinical and Translational Science Award (CTSA). He has published more than 370 peer-reviewed papers addressing the design and analysis of clinical trials and epidemiological studies as well as the evaluation of repeated measurements and correlated data.
Moonseong Heo, PhD, is a professor in the Department of Epidemiology & Population Health at the Albert Einstein College of Medicine. His research includes sample size determinations for clinical trials, meta-analysis, longitudinal data analysis applying mixed-effects models, handling attrition problems in clinical trials data, and epidemiology in the fields of obesity and psychiatry.
Song Zhang, PhD, is an associate professor in the Department of Clinical Sciences at the University of Texas Southwestern Medical Center. He has extensive experience in the design of clinical trials with correlated outcomes, addressing challenges that involve different correlation structures, missing data patterns, financial constraints, and historical controls. He is also interested in Bayesian statistical methods and their application in longitudinal and survival data analysis, high-throughput data analysis, disease mapping, adaptive design for clinical trials, and missing data imputation.
These books may interest you
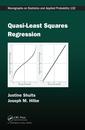
Quasi-Least Squares Regression 184.47 €