Description
Preventing and Treating Missing Data in Longitudinal Clinical Trials
A Practical Guide
Practical Guides to Biostatistics and Epidemiology Series
Author: Mallinckrodt Craig H.
Focuses on the prevention and treatment of missing data in longitudinal clinical trials, looking at key principles and explaining analytic methods.
Language: English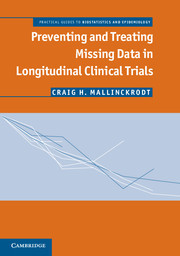
Subject for Preventing and Treating Missing Data in Longitudinal...:
Approximative price 35.19 €
In Print (Delivery period: 14 days).
Add to cart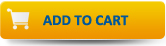
Preventing and Treating Missing Data in Longitudinal Clinical Trials
Publication date: 01-2013
180 p. · 17.4x24.7 cm · Paperback
Publication date: 01-2013
180 p. · 17.4x24.7 cm · Paperback
Preventing and Treating Missing Data in Longitudinal Clinical Trials
Publication date: 01-2013
180 p. · 18x25.5 cm · Hardback
Publication date: 01-2013
180 p. · 18x25.5 cm · Hardback
Description
/li>Contents
/li>Biography
/li>
Recent decades have brought advances in statistical theory for missing data, which, combined with advances in computing ability, have allowed implementation of a wide array of analyses. In fact, so many methods are available that it can be difficult to ascertain when to use which method. This book focuses on the prevention and treatment of missing data in longitudinal clinical trials. Based on his extensive experience with missing data, the author offers advice on choosing analysis methods and on ways to prevent missing data through appropriate trial design and conduct. He offers a practical guide to key principles and explains analytic methods for the non-statistician using limited statistical notation and jargon. The book's goal is to present a comprehensive strategy for preventing and treating missing data, and to make available the programs used to conduct the analyses of the example dataset.
Part I. Background and Setting: 1. Why missing data matter; 2. Missing data mechanisms; 3. Estimands; Part II. Preventing Missing Data: 4. Trial design considerations; 5. Trial conduct considerations; Part III. Analytic Considerations: 6. Methods of estimation; 7. Models and modeling considerations; 8. Methods of dealing with missing data; Part IV. Analyses and the Analytic Road Map: 9. Analyses of incomplete data; 10. MNAR analyses; 11. Choosing primary estimands and analyses; 12. The analytic road map; 13. Analyzing incomplete categorical data; 14. Example; 15. Putting principles into practice.
Craig H. Mallinckrodt is Research Fellow in the Decision Sciences and Strategy Group at Eli Lilly and Company. Dr Mallinckrodt has supported drug development in all four clinical phases and in several therapeutic areas. He currently leads Lilly's Advanced Analytics hub for missing data and their Placebo Response Task Force, and is a member of a number of other scientific work groups. He has authored more than 170 papers, book chapters and texts, including extensive works on missing data and longitudinal data analysis in journals such as Statistics in Medicine, Pharmaceutical Statistics, the Journal of Biopharmaceutical Statistics, the Journal of Psychiatric Research, the Archives of General Psychiatry, and Nature. He currently chairs the Drug Information Association's Scientific Working Group on Missing Data.
© 2024 LAVOISIER S.A.S.