Description
Real-Time Data Analytics for Large Scale Sensor Data
Advances in ubiquitous sensing applications for healthcare Series
Coordinators: Das Himansu, Dey Nilanjan, Balas Valentina Emilia
Language: English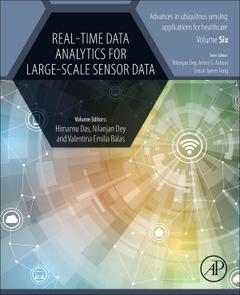
Subjects for Real-Time Data Analytics for Large Scale Sensor Data:
298 p. · 19x23.3 cm · Paperback
Description
/li>Contents
/li>Biography
/li>Comment
/li>
Real-Time Data Analytics for Large-Scale Sensor Data covers the theory and applications of hardware platforms and architectures, the development of software methods, techniques and tools, applications, governance and adoption strategies for the use of massive sensor data in real-time data analytics. It presents the leading-edge research in the field and identifies future challenges in this fledging research area. The book captures the essence of real-time IoT based solutions that require a multidisciplinary approach for catering to on-the-fly processing, including methods for high performance stream processing, adaptively streaming adjustment, uncertainty handling, latency handling, and more.
1. Internet of Things in healthcare: Smart devices, sensors, and systems related to diseases and health conditions 2. Real-time data analytics in healthcare using the Internet of Things 3. Lightweight code self-verification using return-oriented programming in resilient IoT 4. Monte-Carlo Simulation models for reliability analysis of low-cost IoT communication networks in smart grid 5. Lightweight ciphertext-policy attribute-based encryption scheme for data privacy and security in cloud-assisted IoT 6. Soft sensor with shape descriptors for flame quality prediction based on LSTM regression 7. Communication-aware edge-centric knowledge dissemination in edge computing environments 8. An effective blockchain-based, decentralized application for smart building system management 9. Privacy and security of Internet of Things devices 10. Software-Defined Networking for the Internet of Things: Securing home networks using SDN
Nilanjan Dey is an Associate Professor in the Department of Computer Science and Engineering, Techno International New Town, Kolkata, India. He is a visiting fellow of the University of Reading, UK. He also holds a position of Adjunct Professor at Ton Duc Thang University, Ho Chi Minh City, Vietnam. Previously, he held an honorary position of Visiting Scientist at Global Biomedical Technologies Inc., CA, USA (2012–2015). He was awarded his PhD from Jadavpur University in 2015. He is the Editor-in-Chief of the International Journal of Ambient Computing and Intelligence , IGI Global, USA. He is the Series Co-Editor of Springer Tracts in Nature-Inspired Computing (SpringerNature), Data-Intensive Research(SpringerNature), Advances in Ubiquitous Sensing Applications for Healthcare (Elsevier). He was an associate editor of IET Image Processing and editorial board member of Complex & Intelligent Systems, Springer Nature. He is an editorial board member of
- Examines IoT applications, the design of real-time intelligent systems, and how to manage the rapid growth of the large volume of sensor data
- Discusses intelligent management systems for applications such as healthcare, robotics and environment modeling
- Provides a focused approach towards the design and implementation of real-time intelligent systems for the management of sensor data in large-scale environments