Description
A Guide to Simulation (2nd Ed., Softcover reprint of the original 2nd ed. 1987)
Authors: Bratley Paul, Fox Bennet L., Schrage Linus E.
Language: English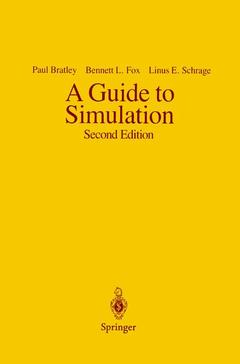
Subjects for A Guide to Simulation:
Keywords
Approximation; Monte Carlo; STATISTICA; linear optimization; model; performance; simulation
158.24 €
In Print (Delivery period: 15 days).
Add to cart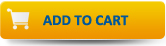
Publication date: 10-2012
397 p. · 15.5x23.5 cm · Paperback
397 p. · 15.5x23.5 cm · Paperback
Description
/li>Contents
/li>
Changes and additions are sprinkled throughout. Among the significant new features are: ? Markov-chain simulation (Sections 1. 3, 2. 6, 3. 6, 4. 3, 5. 4. 5, and 5. 5); ? gradient estimation (Sections 1. 6, 2. 5, and 4. 9); ? better handling of asynchronous observations (Sections 3. 3 and 3. 6); ? radically updated treatment of indirect estimation (Section 3. 3); ? new section on standardized time series (Section 3. 8); ? better way to generate random integers (Section 6. 7. 1) and fractions (Appendix L, program UNIFL); ? thirty-seven new problems plus improvements of old problems. Helpful comments by Peter Glynn, Barry Nelson, Lee Schruben, and Pierre Trudeau stimulated several changes. Our new random integer routine extends ideas of Aarni Perko. Our new random fraction routine implements Pierre L'Ecuyer's recommended composite generator and provides seeds to produce disjoint streams. We thank Springer-Verlag and its late editor, Walter Kaufmann-Bilhler, for inviting us to update the book for its second edition. Working with them has been a pleasure. Denise St-Michel again contributed invaluable text-editing assistance. Preface to the First Edition Simulation means driving a model of a system with suitable inputs and observing the corresponding outputs. It is widely applied in engineering, in business, and in the physical and social sciences.
1 Introduction.- 1.1. Systems, Models, and Simulation.- 1.2. Verification, Approximation, and Validation.- 1.2.1. Verifying a Program.- 1.2.2. Approximation and Validation.- 1.3. States, Events, and Clocks.- 1.4. Simulation—Types and Examples.- 1.4.1. Synchronous and Asynchronous Discrete-Event Simulation.- 1.4.2. Continuous Simulation.- 1.4.3. Hybrid Simulation.- 1.5. Introduction to Random Numbers.- 1.6. Perspective on Experimental Design and Estimation.- 1.7. Clock Mechanisms.- 1.8. Hints for Simulation Programming.- 1.9. Miscellaneous Problems.- 2 Variance Reduction.- 2.1. Common Random Numbers.- 2.1.1. Informal Approach.- 2.1.2. Formal Development.- 2.1.3. Auxiliary Results.- 2.2. Antithetic Variates.- 2.3. Control Variates.- 2.4. Stratification.- 2.5. Importance Sampling.- 2.6. Conditional Monte Carlo.- 2.7. Jackknifing.- 3 Output Analysis.- 3.1. Introduction.- 3.1.1. Finite-Horizon Versus Steady-State Performance.- 3.1.2. Fixed Sample Size Versus Sequential Sampling.- 3.2. Analysis of Finite-Horizon Performance.- 3.2.1. Absolute Performance Estimation.- 3.2.2. Relative Performance Estimation.- 3.3. Analysis of Steady-State Performance.- 3.3.1. Batch Means.- 3.3.2. Regenerative Methods.- 3.3.3. Spectral Analysis Methods.- 3.3.4. Autoregressive Methods.- 3.3.5. Recommendations.- 3.4. Analysis of Transaction-Based Performance.- 3.5. Indirect Estimation via r = ?s.- 3.6. Problems.- 3.7. Renewal Theory Primer.- 3.8. Standardized Time Series.- 3.8.1. Steady State.- 3.8.2. Transients.- 4 Rational Choice of Input Distributions.- 4.1. Addition and the Normal Distribution.- 4.2. Multiplication and the Lognormal.- 4.3. Memorylessness and the Exponential.- 4.4. Superposition, the Poisson, and the Exponential.- 4.5. Minimization and the Weibull Distribution.- 4.6. A Mixed Empirical and Exponential Distribution.- 4.7. Extreme Values and Spacings.- 4.8. When Not to Use a Theoretical Distribution.- 4.9. Nonstationary Poisson Processes.- 5 Nonuniform Random Numbers.- 5.1. Introduction.- 5.2. General Methods.- 5.2.1. Inversion.- 5.2.2. Tabular Approximation of the Inverse Transform.- 5.2.3. Empirical cdf’s.- 5.2.4. A Mixed Empirical and Exponential Distribution.- 5.2.5. Rejection.- 5.2.6. Generalized Rejection.- 5.2.7. Composition.- 5.2.8. The Alias Method for Discrete Distributions.- 5.2.9. Functional Approximations of the Inverse Transform.- 5.2.10. Other Ingenious Methods.- 5.3. Continuous Distributions.- 5.3.1. The Nonstandard Normal Distribution.- 5.3.2. The Multivariate (Dependent) Normal Distribution.- 5.3.3. Symmetric Stable Variates.- 5.3.4. The Cauchy Distribution.- 5.3.5. The Lognormal Distribution.- 5.3.6. The Exponential Distribution.- 5.3.7. The Hyperexponential Distribution.- 5.3.8. The Laplace and Exponential Power Distributions.- 5.3.9. Erlang and Gamma Distributions.- 5.3.10. The Beta Distribution.- 5.3.11. The Chi-square Distribution.- 5.3.12. The F-Distribution.- 5.3.13. The t-Distribution.- 5.3.14. The Weibull Distribution.- 5.3.15. The Gumbel Distribution.- 5.3.16. The Logistic Distribution.- 5.3.17. The Generalized Lambda Distribution.- 5.3.18. Nonhomogeneous Poisson Processes.- 5.4. Discrete Distributions.- 5.4.1. The Binomial Distribution.- 5.4.2. The Poisson Distribution.- 5.4.3. Compound Poisson Distributions.- 5.4.4. The Hypergeometric Distribution.- 5.4.5. The Geometric Distribution.- 5.4.6. The Negative Binomial and Pascal Distributions.- 5.4.7. Multidimensional Poisson Distributions.- 5.5. Problems.- 5.6. Timings.- 6 Uniform Random Numbers.- 6.1. Random Introductory Remarks.- 6.2. What Constitutes Randomness.- 6.3. Classes of Generators.- 6.3.1. Random Devices.- 6.3.2. Tables.- 6.3.3. The Midsquare Method.- 6.3.4. Fibonacci and Additive Congruential Generators.- 6.3.5. Linear Congruential Generators.- 6.3.6. Linear Recursion mod 2 Generators.- 6.3.7. Combinations of Generators.- 6.4. Choosing a Good Generator Based on Theoretical Considerations.- 6.4.1. Serial Correlation of Linear Congruential Generators.- 6.4.2. Cycle Length of Linear Congruential Generators.- 6.4.3. Cycle Length for Tausworthe Generators.- 6.4.4. The Spectral Test.- 6.5. Implementation of Uniform Random Number Generators.- 6.5.1. Multiplicative Generator With Modulus 2.- 6.5.2. Multiplicative Generators With Prime Modulus.- 6.5.3. Implementing the Tausworthe Generator.- 6.6. Empirical Testing of Uniform Random Number Generators.- 6.6.1. Chi-square Tests.- 6.6.2. Kolmogorov-Smirnov Tests.- 6.6.3. Tests Specifically for Uniform Random Number Sequences.- 6.7. Proper Use of a Uniform Random Number Generator.- 6.7.1. Generating Random Integers Uniform Over an Arbitrary Interval.- 6.7.2. Nonrandomness in the Low-order Bits of Multiplicative Generators.- 6.7.3. Linear Congruential Generators and the Box-Muller Method.- 6.8. Exploiting Special Features of Uniform Generators.- 6.8.1. Generating Antithetic Variates With a Multiplicative Congruential Generator.- 6.8.2. Generating a Random Number Stream in Reverse Order.- 6.8.3. Generating Disjoint Sequences.- 7 Simulation Programming.- 7.1. Simulation With General-Purpose Languages.- 7.1.1. The Simplest Possible Clock Mechanism.- 7.1.2. Generating Random Variates.- 7.1.3. Data Structures in Fortran.- 7.1.4. A Complete Simulation in Fortran.- 7.1.5. Fortran Simulation Packages.- 7.1.6. Our Standard Example—the Naive Approach.- 7.1.7. Simulation Using Pascal.- 7.2. Simscript.- 7.2.1. Data Structures in Simscript.- 7.2.2. Simscript and Simulation.- 7.2.3. A Complete Simulation in Simscript.- 7.2.4. The Standard Example in Simscript.- 7.2.5. Processes and Resources.- 7.2.6. Summing-up.- 7.3. GPSS.- 7.3.1. The Basic Concepts.- 7.3.2. Resources in GPSS.- 7.3.3. Generating Random Variates.- 7.3.4. A Complete Simulation in GPSS.- 7.3.5. The Standard Example in GPSS.- 7.3.6. Summing-up.- 7.4. Simula.- 7.4.1. The Class Concept in Simula.- 7.4.2. Simulation Using System Classes and Procedures.- 7.4.3. A Complete Simulation in Simula.- 7.4.4. Demos.- 7.4.5. The Standard Example in Simula With Demos.- 7.4.6. Summing-up.- 7.5. General Considerations in Simulation Programming.- 7.5.1. Language Design.- 7.5.2. System Considerations in Simulation.- 7.5.3. Statistical Considerations.- 8 Programming to Reduce the Variance.- 8.1. Choosing an Input Distribution.- 8.1.1. Using an Exact Method.- 8.1.2. Inverting a Tabulated Distribution.- 8.1.3. Using a Mixed Empirical and Exponential Distribution.- 8.1.4. Testing Robustness.- 8.2. Common Random Numbers.- 8.3. Antithetic Variates.- 8.4. Control Variates.- 8.4.1. The Simple Approach.- 8.4.2. Regression With Splitting.- 8.4.3. Regression With Jackknifing.- 8.5. Stratified Sampling.- 8.6. Importance Sampling.- 8.7. Conditional Monte Carlo.- 8.8. Summary.- Appendix A The Shapiro—Wilk Test for Normality.- Appendix L Routines for Random Number Generation.- Appendix X Examples of Simulation Programming.- References.- Author Index.
© 2024 LAVOISIER S.A.S.
These books may interest you
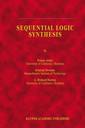
Sequential Logic Synthesis 105.49 €