Description
Applied Hierarchical Modeling in Ecology: Analysis of distribution, abundance and species richness in R and BUGS
Volume 1:Prelude and Static Models
Authors: Kéry Marc, Royle J. Andrew
Language: English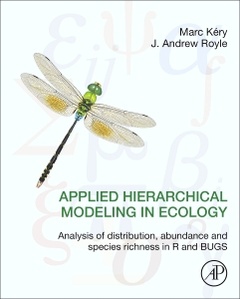
Subjects for Applied Hierarchical Modeling in Ecology: Analysis of...:
Keywords
Abundance; Availability; Bayesian inference; Bayesian p-value; Binomial; Bootstrap; Bootstrapping; BUGS; Capture-recapture sampling; Community N-mixture model; Community occupancy model; Community; Conditional likelihood; Conditional probability; Coverage bias; Dail�Madsen model; Data augmentation; Data simulation; Delta method; Density; Detectability; Detection probability; Detection; Distance sampling; Distribution; Double observer protocol; Double-observer sampling; Ecological process; Effective sample area; False-negative error; False-negative; False-positive error; False-positive; Fixed effects; Full likelihood; Generalized linear mixed model; Generalized linear model (GLM)Goodness of fit; Generalized linear model; Gibbs sampling; GLM; GLMM; Group size; Hierarchical distance sampling; Hierarchical model; Hierarchical models; Integrated likelihood; Island scrub jay; JAGS; Joint species distribution model; JSDM; Least-squares; Line transect; Linear model; Linear predictor; Link function; LMM; Marginal likelihood; Markov chain Monte Carlo (MCMC)Mixed model; Markov chain Monte Carlo; Maximum likelihood; Measurement error; Metacommunity; Metropolis�Hastings; Missing value; Mixed model; Multinomial distribution; Multispecies abundance model; Multispecies occupancy model; Negative binomial; N-mixture model; Nominal sample area; Observation process; Occupancy; Occurrence; OpenBUGS; Parameterization; Parametric bootstrap; Point process; Point transect; Poisson�lognormal; Poisson; Population dynamics; Posterior distribution; Posterior predictive distribution; Prediction; Presence/Absence; Presence/absence; R2; Random effects; Random-effects model; Removal sampling; Robust design; Spatial distance sampling; Species accumulation; Species distribution model; Species richness; State-space model; Sunflower effect; Survival probability; Temporary emigration; Time removal; Unmarked; Vital rates; Wagtail; WinBUGS; Zero inflation; Zero-inflated Poisson
808 p. · 19x23.3 cm · Hardback
Description
/li>Contents
/li>Biography
/li>Comment
/li>
Applied Hierarchical Modeling in Ecology: Distribution, Abundance, Species Richness offers a new synthesis of the state-of-the-art of hierarchical models for plant and animal distribution, abundance, and community characteristics such as species richness using data collected in metapopulation designs. These types of data are extremely widespread in ecology and its applications in such areas as biodiversity monitoring and fisheries and wildlife management.
This first volume explains static models/procedures in the context of hierarchical models that collectively represent a unified approach to ecological research, taking the reader from design, through data collection, and into analyses using a very powerful class of models. Applied Hierarchical Modeling in Ecology,Volume 1 serves as an indispensable manual for practicing field biologists, and as a graduate-level text for students in ecology, conservation biology, fisheries/wildlife management, and related fields.
Preface
Part 1: Prelude
1. Distribution, abundance and species richness in ecology
2. What are hierarchical models and how do we analyse them ?
3. Linear models, generalized linear models (GLMs), and random-effects: the components of hierarchical models
4. Introduction to data simulation
5. The Bayesian modeling software BUGS and JAGS
Part 2: Models for static systems
6. Modeling abundance using binomial N-mixture models
7. Modeling abundance using multinomial N-mixture models
8. Modeling abundance using hierarchical distance sampling
9. Advanced hierarchical distance sampling
10. Modeling distribution and occurrence using site-occupancy models
11. Community models (incidence- and abundance-based)
Dr Royle is a Senior Scientist and Research Statistician at the U.S. Geological Survey's Patuxent Wildlife Research Center. His research is focused on the application of probability and statistics to ecological problems, especially those related to animal sampling and demographic modeling. Much of his research over the last 10 years has been devoted to the development of methods illustrated in our new book. He has authored or coauthored more than 100 journal articles, and co-authored the books Spatial Capture Recapture, Hierarchical Modeling and Inference in Ecology and Occupancy Estimation and Modeling: Inferring Patterns and Dynamics of Species Occurrence, all published by Academic Press.
- Provides a synthesis of important classes of models about distribution, abundance, and species richness while accommodating imperfect detection
- Presents models and methods for identifying unmarked individuals and species
- Written in a step-by-step approach accessible to non-statisticians and provides fully worked examples that serve as a template for readers' analyses
- Includes companion website containing data sets, code, solutions to exercises, and further information