Description
Machine Learning Methods for Behaviour Analysis and Anomaly Detection in Video, 1st ed. 2018
Springer Theses Series
Language: English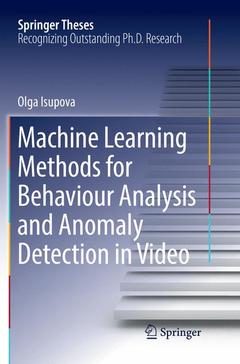
Subject for Machine Learning Methods for Behaviour Analysis and...:
Publication date: 01-2019
126 p. · 15.5x23.5 cm · Paperback
Publication date: 03-2018
Support: Print on demand
Description
/li>Contents
/li>Comment
/li>
This thesis proposes machine learning methods for understanding scenes via behaviour analysis and online anomaly detection in video. The book introduces novel Bayesian topic models for detection of events that are different from typical activities and a novel framework for change point detection for identifying sudden behavioural changes.
Behaviour analysis and anomaly detection are key components of intelligent vision systems. Anomaly detection can be considered from two perspectives: abnormal events can be defined as those that violate typical activities or as a sudden change in behaviour. Topic modelling and change-point detection methodologies, respectively, are employed to achieve these objectives.
The thesis starts with the development of learning algorithms for a dynamic topic model, which extract topics that represent typical activities of a scene. These typical activities are used in a normality measure in anomaly detection decision-making. The book also proposes anovel anomaly localisation procedure.In the first topic model presented, a number of topics should be specified in advance. A novel dynamic nonparametric hierarchical Dirichlet process topic model is then developed where the number of topics is determined from data. Batch and online inference algorithms are developed.
The latter part of the thesis considers behaviour analysis and anomaly detection within the change-point detection methodology. A novel general framework for change-point detection is introduced. Gaussian process time series data is considered. Statistical hypothesis tests are proposed for both offline and online data processing and multiple change point detection are proposed and theoretical properties of the tests are derived.
The thesis is accompanied by open-source toolboxes that can be used by researchers and engineers.