Description
Nonlinear Time Series
Theory, Methods and Applications with R Examples
Chapman & Hall/CRC Texts in Statistical Science Series
Authors: Douc Randal, Moulines Eric, Stoffer David
Language: English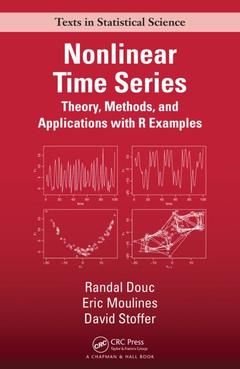
Subjects for Nonlinear Time Series:
Keywords
Sample ACF; Linear Models; Asymmetric Power Arch; Linear Gaussian State Space Models; Stochastic Recurrence Equations; Beyond Linear Models; Comprehensive Nuclear Test Ban Treaty; ARMA Type Model; Markov Models; Basic Stochastic Volatility Model; Markovian Models; BLP; ARMA Model; Conditional Expectation; EEG Trace; Overdispersed Poisson Model; Strong White Noise; GARCH Model; Negative Binomial; Order Volterra; Stochastic Volatility Model; Higher Order Spectra; ARMA Coefficient; Linear Gaussian Process; Arch Model; Volterra Series; Tar Model
135.96 €
In Print (Delivery period: 15 days).
Add to cart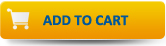
530 p. · 15.6x23.4 cm · Hardback
Description
/li>Contents
/li>Readership
/li>Biography
/li>
Designed for researchers and students, Nonlinear Times Series: Theory, Methods and Applications with R Examples familiarizes readers with the principles behind nonlinear time series models?without overwhelming them with difficult mathematical developments. By focusing on basic principles and theory, the authors give readers the background required to craft their own stochastic models, numerical methods, and software. They will also be able to assess the advantages and disadvantages of different approaches, and thus be able to choose the right methods for their purposes.
The first part can be seen as a crash course on "classical" time series, with a special emphasis on linear state space models and detailed coverage of random coefficient autoregressions, both ARCH and GARCH models. The second part introduces Markov chains, discussing stability, the existence of a stationary distribution, ergodicity, limit theorems, and statistical inference. The book concludes with a self-contained account on nonlinear state space and sequential Monte Carlo methods. An elementary introduction to nonlinear state space modeling and sequential Monte Carlo, this section touches on current topics, from the theory of statistical inference to advanced computational methods.
The book can be used as a support to an advanced course on these methods, or an introduction to this field before studying more specialized texts. Several chapters highlight recent developments such as explicit rate of convergence of Markov chains and sequential Monte Carlo techniques. And while the chapters are organized in a logical progression, the three parts can be studied independently.
Statistics is not a spectator sport, so the book contains more than 200 exercises to challenge readers. These problems strengthen intellectual muscles strained by the introduction of new theory and go on to extend the theory in significant ways. The book helps readers hone their skills in nonlinear time series analysis and their applications.
Preliminaries. Markov and Iterative Models: Nonlinear Markovian Models. Stability, Recurrence, Mixing. Ergodicity, Limit Theorems. Parametric Inference. Nonparametric Inference. Hidden Markov Models: Some HMM Models. Filtering and Smoothing in HMM. Parametric Inference for HMM. Nonparametric Inference for HMM. Particle Filtering Basics. Advanced Issues in Particle Filtering. Particle Smoothing Basics. Numerical Methods for Inference.