Performance Analysis and Modeling of Digital Transmission Systems, Softcover reprint of the original 1st ed. 2004 Information Technology: Transmission, Processing and Storage Series
Langue : Anglais
Auteur : Turin William
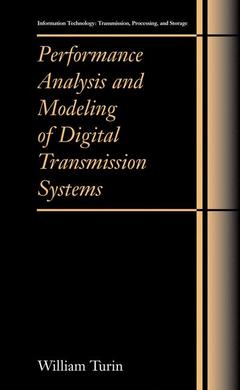
This book is an expanded third edition of the book Performance Analysis of Digital Transmission Systems, originally published in 1990. Second edition of the book titled Digital Transmission Systems: Performance Analysis and Modeling was published in 1998. The book is intended for those who design communication systems and networks. A computer network designer is interested in selecting communication channels, error protection schemes, and link control protocols. To do this efficiently, one needs a mathematical model that accurately predicts system behavior. Two basic problems arise in mathematical modeling: the problem of identifying a system and the problem of applying a model to the system analysis. System identification consists of selecting a class of mathematical objects to describe fundamental properties of the system behavior. We use a specific class of hidden Markov models (HMMs) to model communication systems. This model was introduced by C. E. Shannon more than 50 years ago as a Noisy Discrete Channel with a finite number of states. The model is described by a finite number of matrices whose elements are estimated on the basis of experimental data. We develop several methods of model identification and show their relationship to other methods of data analysis, such as spectral methods, autoregressive moving average CARMA) approximations, and rational transfer function approximations.
Preface.- Notation.- 1. Error Source Models.- 1.1 Description of Error Sources by Hidden Markov Models.- 1.1.1 Finite State Channel.- 1.1.2 Hidden Markov Models.- 1.1.3 Discrete-Time Finite State Systems.- 1.1.4 Error Source HMM.- 1.1.5 Gilbert’s Model.- 1.1.6 Equivalent Models.- 1.1.7 The HMM Model Generality.- 1.1.8 Satellite Channel Model.- 1.1.9 Fading Wireless Channels.- 1.1.10 Concatenated Channel Error Sources.- 1.2 Binary Symmetric Stationary Channel.- 1.2.1 Model Description.- 1.2.2 Diagonalization of the Matrices.- 1.2.3 Models with Two Sets of States.- 1.2.4 Block Matrix Representation.- 1.3 Error Source Description by Matrix Processes.- 1.3.1 Matrix Process Definition.- 1.3.2 Block Matrix Representation.- 1.3.3 Matrix Processes and Difference Equations.- 1.3.4 Matrix Processes and Markov Functions.- 1.4 Error Source Description by Semi-Markov Processes.- 1.4.1 Semi-Markov Processes.- 1.4.2 Semi-Markov Lumping of Markov Chain.- 1.5 Some Particular Error Source Models.- 1.5.1 Single-Error-State Models.- 1.5.2 Alternating Renewal Process Models.- 1.5.3 Alternating State HMM.- 1.5.4 Fading Channel Errors.- 1.6 Conclusion.- References.- 2. Matrix Probabilities.- 2.1 Matrix Probabilities and Their Properties.- 2.1.1 Basic Definitions.- 2.1.2 Properties of Matrix Probabilities.- 2.1.3 Random Variables.- 2.2 Matrix Transforms.- 2.2.1 Matrix Generating Functions.- 2.2.2 Matrix z-Transforms.- 2.2.3 Matrix Fourier Transform.- 2.2.4 Matrix Discrete Fourier Transform.- 2.2.5 Matrix Transforms and Difference Equations.- 2.3 Matrix Distributions.- 2.3.1 Matrix Multinomial Distribution.- 2.3.2 Matrix Binomial Distribution.- 2.3.3 Matrix Pascal Distribution.- 2.4 Markov Functions.- 2.4.1 Block Matrix Probabilities.- 2.4.2 Matrix Multinomial Distribution.- 2.4.3 Interval Distributions of Markov Functions.- 2.4.4 Signal Flow Graph Applications.- 2.5 Monte Carlo Method.- 2.5.1 Error Source Simulation.- 2.5.2 Performance Characteristic Calculation.- 2.6 Computing Scalar Probabilities.- 2.6.1 The Forward and Backward Algorithms.- 2.6.2 Scalar Generating Functions.- 2.7 Conclusion.- References.- 3. Model Parameter Estimation.- 3.1 The Em Algorithm.- 3.1.1 Kullback-Leibler Divergence.- 3.1.2 Minimization Algorithms.- 3.1.3 The EM Algorithm.- 3.1.4 Maximization of a Function.- 3.1.5 EM Algorithm Acceleration.- 3.1.6 Statistical EM Algorithm.- 3.2 Baum-Welch Algorithm.- 3.2.1 Phase-Type Distribution Approximation.- 3.2.2 HMM Approximation.- 3.2.3 Fitting HMM to Experimental Data.- 3.2.4 Matrix Form of the BWA.- 3.2.5 Scaling.- 3.3 Markov Renewal Process.- 3.3.1 Fast Forward and Backward Algorithms.- 3.3.2 Fitting MRP.- 3.4 Matrix-Geometric Distribution Parameter Estimation.- 3.4.1 Distribution Simplification.- 3.4.2 ML Parameter Estimation.- 3.4.3 Sequential Least Mean Square Method.- 3.4.4 Piecewise Logarithmic Transformation.- 3.4.5 Prony’s Method.- 3.4.6 Matrix Echelon Parametrization.- 3.4.7 Utilization of the Cumulative Distribution Function.- 3.4.8 Method of Moments.- 3.4.9 Transform Domain Approximation.- 3.5 Matrix Process Parameter Estimation.- 3.5.1 ML Parameter Estimation.- 3.5.2 Interval Distribution Estimation.- 3.5.3 Utilization of Two-Dimensional Distributions.- 3.5.4 Polygeometric Distributions.- 3.5.5 Error Bursts.- 3.6 Hmm Parameter Estimation.- 3.6.1 Multilayered Error Clusters.- 3.6.2 Nested Markov Chains.- 3.6.3 Single-Error-State Models.- 3.7 Monte Carlo Method of Model Building.- 3.8 Error Source Model in Several Channels.- 3.9 Conclusion.- References.- 4. Performance of Forward Error-Correction Systems.- 4.1 Basic Characteristics of One-Way Systems.- 4.2 Elements of Error-Correcting Coding.- 4.2.1 Field Galois Arithmetic.- 4.2.2 Linear Codes.- 4.2.3 Cyclic Codes.- 4.2.4 Bose-Chaudhuri-Hocquenghem Codes.- 4.2.5 Reed-Solomon Codes.- 4.2.6 Convolutional Codes.- 4.2.6 Trellis-Code Modulation.- 4.3 Maximum A Posteriori Decoding.- 4.3.1 MAP Symbol Estimation.- 4.3.2 MAP Sequence Decoding.- 4.4 Block Code Performance Characterization.- 4.4.1 The Probability of Undetected Error.- 4.4.2 Performance of Forward Error-Correcting Codes.- 4.4.3 Symmetrically Dependent Errors.- 4.4.4 Upper and Lower Bounds.- 4.4.5 Postdecoding Error Probability.- 4.4.6 Soft Decision Decoding.- 4.4.7 Correcting Errors and Erasures.- 4.4.8 Product Codes.- 4.4.9 Concatenated Codes.- 4.5 Convolutional Code Performance.- 4.5.1 Viterbi Algorithm Performance.- 4.5.2 Syndrome Decoding Performance.- 4.6 Computer Simulation.- 4.7 Zero-Redundancy Codes.- 4.7.1 Interleaving.- 4.7.2 Encryptors and Scramblers.- 4.8 Conclusion.- References.- 5. Performance Analysis of Communication Protocol.- 5.1 Basic Characteristics of Two-Way Systems.- 5.2 Return-Channel Messages.- 5.3 Synchronization.- 5.4 Arq Performance Characteristics.- 5.4.1 Stop-and-Wait ARQ.- 5.4.2 Message Delay Distribution.- 5.4.3 Insertion and Loss Interval Distribution.- 5.4.4 Accepted Messages.- 5.4.5 Alternative Assumptions.- 5.4.6 Modified Stop-and-Wait ARQ.- 5.4.7 Go-Back-N ARQ.- 5.4.8 Multiframe Messages.- 5.5 Delay-Constained Systems.- 5.5.1 Queue-Length Probability Distribution.- 5.5.2 Packet Delay Probability.- 5.6 Conclusion.- References.- 6. Continuous Time Hmm.- 6.1 Continuous and Discrete Time Hmm.- 6.1.1 Markov Arrival Processes.- 6.1.2 MAP Discrete Skeleton.- 6.1.3 Matrix Poisson Distribution.- 6.2 Fitting Continuous Time Hmm.- 6.2.1 Fitting Phase-Type Distribution.- 6.2.2 HMM Approximation.- 6.3 Conclusion.- References.- 7. Continuous State Hmm.- 7.1 Continuous and Discrete State Hmm.- 7.2 Operator Probability.- 7.3 Filtering, Prediction, and Smoothing.- 7.4 Linear Systems.- 7.4.1 Autocovariance Function.- 7.4.2 Observation Sequence PDF.- 7.4.3 Kalman Filter PDF.- 7.4.4 The Innovation Representation PDF.- 7.4.5 The Backward Algorithm.- 7.4.6 The Forward-Backward Algorithm PDF.- 7.4.7 RTS Smoother.- 7.4.8 Viterbi Algorithm.- 7.5 Autoregressive Moving Average Processes.- 7.5.1 State Space Representation.- 7.5.2 Autocovariance Function.- 7.5.3 The Forward Algorithm.- 7.5.4 RTS Smoother.- 7.6 Parameter Estimation.- 7.6.1 HMM Approximation.- 7.6.2 HGMM Approximation.- 7.7 Arma Channel Modeling.- 7.7.1 Fading Channel.- 7.7.2 Ultra-Wide Bandwidth Channel.- 7.8 Conclusion.- References.- Appendix 1.- 1.1 Matrix Processes.- 1.1.1 Reduced Canonic Representation.- 1.2 Markov Lumpable Chains.- 1.3 Semi-Markov Lumpable Chains.- References.- Appendix 2.- 2.1 Asymptotic Expansion of Matrix Probabilities.- 2.2 Chernoff Bounds.- 2.3 Block Graphs.- References.- Appendix 3.- 3.1 Statistical Inference.- 3.1.1 Distribution Parameter Estimation.- 3.1.2 Hypothesis Testing.- 3.1.3 Goodness of Fit.- 3.1.4 Confidence Limits.- 3.2 Markov Chain Model Building.- 3.2.1 Statistical Tests for Simple Markov Chains.- 3.2.2 Multiple Markov Chains.- 3.3 Semi-Markov Process Hypothesis Testing.- 3.3.1 ML Parameter Estimation.- 3.3.2 Grouped Data.- 3.3.3 Underlying Markov Chain Parameter Estimation.- 3.3.4 Autonomous Semi-Markov Processes.- 3.4 Matrix Process Parameter Estimation.- References.- Appendix 4.- 4.1 Sums With Binomial Coefficients.- 4.2 Maximum-Distance-Separable Code Weight Generating Function.- 4.3 Union Bounds on Viterbi Algorithm Performance.- References.- Appendix 5.- 5.1 Matrices.- 5.1.1 Basic Definitions.- 5.1.2 Systems of Linear Equations.- 5.1.3 Calculating Powers of a Matrix.- 5.1.4 Eigenvectors and Eigenvalues.- 5.1.5 Similar Matrices.- 5.1.6 Matrix Series Summation.- 5.1.7 Product of Several Matrices.- 5.1.8 Fast Exponentiation.- 5.1.9 Matrix Derivatives.- 5.1.10 Quadratic Polynomials.- 5.1.11 Quadratic Forms.- References.- Appendix 6.- 6.1 Markov Chains and Graphs.- 6.1.1 Transition Probabilities.- 6.1.2 Stationary Markov Chains.- 6.1.3 Partitioning States of a Markov Chains.- 6.1.4 Signal Flow Graphs.- References.- Appendix 7.- 7.1 Markov Processes.- 7.1.1 Transition Probability Densities.- 7.1.2 Transition Probability Operators.- 7.2 Gauss-Markov Processes.- 7.2.1 Gaussian Random variables and Processes.- 7.2.2 Linear Systems.- 7.2.3 Operator Pm.- 7.2.4 Stationary Distribution.- 7.2.5 Autocovariance Function.- 7.2.6 Autoregressive Processes.- 7.2.7 Parameter Estimation.- References.
Date de parution : 05-2013
Ouvrage de 441 p.
17.8x25.4 cm
Disponible chez l'éditeur (délai d'approvisionnement : 15 jours).
Prix indicatif 52,74 €
Ajouter au panierDate de parution : 06-2004
Ouvrage de 458 p.
Thèmes de Performance Analysis and Modeling of Digital... :
© 2024 LAVOISIER S.A.S.