Description
Robustness in Statistical Forecasting, Softcover reprint of the original 1st ed. 2013
Author: Kharin Yuriy
Language: English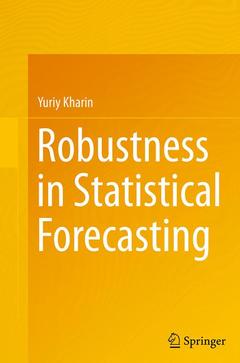
Subjects for Robustness in Statistical Forecasting:
Keywords
62-02, 62M20, 62M10, 62G35, 62-07, 62F35, 62C20, 62P20, forecasting, model distortion, risk, robustness, time series
Approximative price 52.74 €
In Print (Delivery period: 15 days).
Add to cart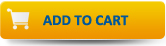
Publication date: 08-2016
Support: Print on demand
Approximative price 52.74 €
In Print (Delivery period: 15 days).
Add to cart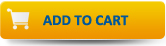
Publication date: 09-2013
356 p. · 15.5x23.5 cm · Hardback
Description
/li>Contents
/li>Biography
/li>Comment
/li>
The first book with a specific focus on robustness of time series forecasting
Evaluates sensitivity of the forecast risks to distortions and presents new robust forecasting procedures
Presentation of the material follows the pattern “model ? method ? algorithm ? computation results based on simulated / real-world data” ?
Includes supplementary material: sn.pub/extras