Description
Statistical Theory and Inference, Softcover reprint of the original 1st ed. 2014
Author: Olive David J.
Language: English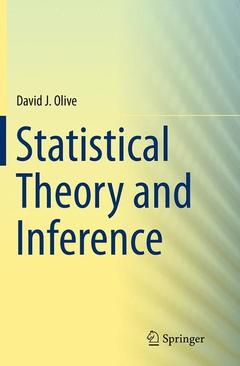
Subject for Statistical Theory and Inference:
Publication date: 09-2016
Support: Print on demand
Publication date: 05-2014
434 p. · 15.5x23.5 cm · Hardback
Description
/li>Contents
/li>Biography
/li>Comment
/li>
This text is for a one semester graduate course in statistical theory and covers minimal and complete sufficient statistics, maximum likelihood estimators, method of moments, bias and mean square error, uniform minimum variance estimators and the Cramer-Rao lower bound, an introduction to large sample theory, likelihood ratio tests and uniformly most powerful tests and the Neyman Pearson Lemma. A major goal of this text is to make these topics much more accessible to students by using the theory of exponential families.
Exponential families, indicator functions and the support of the distribution are used throughout the text to simplify the theory. More than 50 ``brand name" distributions are used to illustrate the theory with many examples of exponential families, maximum likelihood estimators and uniformly minimum variance unbiased estimators. There are many homework problems with over 30 pages of solutions.
Exponential families, indicator functions and the support of the distribution are used throughout the text to simplify the theory
Many homework problems with over 30 pages of solutions
Covers complete sufficient statistics, maximum likelihood estimation, uniformly minimum unbiased estimators, UMP tests, and large sample theory
These books may interest you
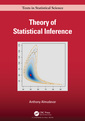
Theory of Statistical Inference 123.78 €